
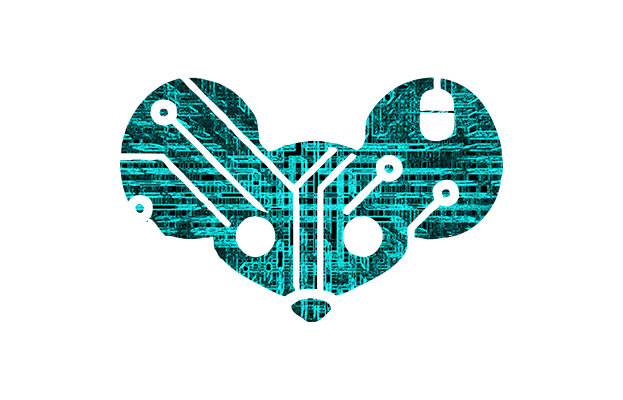
It does indeed. My job includes writing and deploying .NET apps on multiple platforms, and it works fine for me.
But some people prefer not to use .NET when comparable native options are available, so they might prefer KeePassXC.
It does indeed. My job includes writing and deploying .NET apps on multiple platforms, and it works fine for me.
But some people prefer not to use .NET when comparable native options are available, so they might prefer KeePassXC.
Nothing major as far as I can tell. Here’s an overview via SuperUser. KeePassXC might be a better option for some use cases if you’re mostly not on Windows as it does not require .NET. Note that “KeePassXC does not support plugins at the moment and probably never will”, but it does have built-in support for some things you might want a plugin for in KeePass2.
So what will everyone having their own AI look like in a way that doesn’t just sound like a chatGPT joke? What would make it a desirable future?
I guess that depends on how much authority you’d want to delegate.
For me, it would be nice if I could have it do things like shopping tasks without being more of a pain than doing the task myself. For example, I needed to buy a hot-water carpet cleaner today. It would be great if I could tell it
Hey Staffie, buy a carpet shampoo machine for home delivery within the next two weeks. Pick a highly rated machine from a widely recognized brand, and focus on carpet cleaning and water extraction performance, I don’t need any upholstery cleaning features. Don’t spend over $400. If the best option is under $200 don’t ask, just buy it. If it’s over $200, show me the top two options before buying.
And end up with something pretty close to what I’d have picked if I did the shopping myself.
It would also be great if I could have it reliably perform arbitrary tasks that it isn’t specifically programmed to do. Like
Hey Staffie, check if I’ve got enough PTO to take next Thursday and Friday off, and if so, reserve a campsite for me at Foo State Park for three nights, preferably one close to the water, then send Mr. Boss an email letting him know I’ll be out those days.
If it were particularly smart it would infer from previous conversations that I might want a 1lb propane cylinder, marshmallows, graham crackers, and Hershey bars added to my grocery list and would add them automatically (because it already knows my preferences about small automatic expenditures like that and is aware of the spending limits I’ve given it).
Then it might come back a few minutes later and say
'Hey boss, all the campsites within 250 of the water are already reserved, but site 1701D, which is near the only restroom and a tailhead, is available. Reviewers report that the park WiFi access point is installed at the restroom, so that site has good free internet service. Shall I reserve it?
So yeah, in general, the ability to take arbitrary directions and execute them in reasonably intelligent ways (for example If I ask for a site Foo State Park, and there are two such parks in my country, it should be able to guess which park I’m talking about based on the context (like, if I’m reserving 3 nights and one of the parks is an hour down the road and the other is a two day drive, just assume the closer one)) and not require pre-programmed interfaces to every single thing. It should be able to search the web, find the interfaces humans use, and use those to do the kinds of things humans can do. It should also have some capabilities to use my accounts and passwords under a delegated authority to get shit done as my authorized assistant.
Ideally it should also do things like observe my choices and infer my preferences so it can constrain choices it offers me:
Hey Staffie, order lunch from Subway for pickup at 3.
Sure boss, do you want your usual 6 inch turkey sub?
Yep
Nacho cheese chips or salt-n-vinegar?
Nacho.
Done, I’ll let you know when it’s ready.
Stuff like that.
I was curious if that would work on ethernet cable! I’ve seen it done on coax, wasn’t sure if it would work well enough on UTP to be useful outside a lab setting. Cheap too. Cool!
Wire tracker maybe? You might want a higher quality version than that particular one if the cable run is long, one of the reviews suggest that the distance is limited.
That’s what I’ve been wondering too. I keep seeing people complaining about ads, but I use Edge (and Firefox) with Bing regularly on an up-to-date Win 11 system and I’m not seeing anything like that.
Maybe they’ve got demographic targeting that I don’t fit into or something.
I’m not entirely sure how I’m earning Microsoft reward points, but they keep sending me $10 Amazon gift cards for them so… that’s cool anyway.
Yep, two simple mechanical knobs is easily the best microwave oven interface. Although I do like the fancy Samsung microwave I have that is almost completely silent and lightweight, I think it uses an inverter instead of a chonky HV transformer. I wish I could get a combo of that inverter with a couple of simple knobs for controls.
Dry cleaning fluid too!
If I hadn’t already deactivated my account this would have been a good time to do it. I had like 135,000 accounts on my block list.
whatever technical bullshit they needed to do to reverse them
Apparently ultimately this involves hitting the person hiding the encryption keys with a $4 wrench until they provide the keys.
While the outside screen is too thin on my fold
Yep, I’m hoping they’ll do a slightly wider tri-fold model at some point. I’d like to have a wider front screen, like Galaxy S22 Ultra sized, and then be able to unfold twice to get a ~3x sized tablet-sized screen.
Not that that would help with the already astronomical price-tag of the Z-Fold.
ChatGPT currently knows the text of the Harry Potter novels, but it does not recite them when asked to do so.
I tried that several weeks ago while discussing some details of the Harry Potter world with ChatGPT, and it was able to directly quote several passages to me to support its points (we were talking about house elf magic and I asked it to quote a paragraph). I checked against a dead-tree copy of the book and it had exactly reproduced the paragraph as published.
This may have changed with their updates since then, and it may not be able to quote passages reliably, but it is (or was) able to do so on a couple of occasions.
How did you learn to talk to WolframAlpha?
I want to like WA, but the natural language interface is so opaque that I usually give up before I can get any non-trivial calculation out of it.
“Hey, how do I get to the Plex server?”
“Open your browser and go to Hell”
“…”
Previously my server was just a Debian box where I had a ‘docker’ directory with a bunch of .sh files containing ‘docker run’ commands (and a couple of docker-compose files for services that that have closely related containers). That works really well, it’s easy to understand and manage. I had nginx running natively to expose stuff as necessary.
Recently I decided to try TrueNAS Scale (I wanted more reliable storage for my media library, which is large enough to be annoying to replace when a simple drive fails), and I’m still trying to figure it out. It’s kind of a pain in the ass for running containers since the documentation is garbage. The web interface is kind of nice (other than constantly logging me out), but the learning curve for charts and exposing services has been tough, and it seems that ZFS is just a bad choice for Docker.
I was attracted to the idea of being able to run my services on my NAS server as one appliance, but it’s feeling like TrueNAS Scale is way too complicated for home-scale (and way too primitive for commercial, not entirely sure what market they are aiming for) and I’m considering dumping it and setting up two servers, one for NAS and for running my containers and VMs.
For fun I asked ChatGPT about that progressive learning approach, and it seems to like the idea.
I wish I had more time to undertake some experiments in model training, this seems like it would be a really fun research direction.
Sorry for the ‘wall of AI text’:
The idea you’re describing seems to be a form of curriculum learning. In curriculum learning, models are trained in a progressive manner, starting with simple concepts (or in this case, simpler text data) and gradually moving to more complex ones. This approach is motivated by the way human learning often works, where we learn easier topics before moving on to harder ones.
The strategy you’ve suggested, where the model itself determines the complexity or understandability of the next round of training inputs, is interesting. While the traditional approach to curriculum learning is to manually design the learning progression based on human knowledge and intuition, the approach you’re suggesting is more dynamic and autonomous, letting the model guide its own learning process.
As of my last update in September 2021, I can’t confirm any specific projects or papers that have employed exactly this strategy in the domain of large language models. However, there are some related works in the field. For example, some reinforcement learning and computer vision projects have used a similar idea where models adaptively choose their next training samples based on their current understanding. This has been referred to as active learning, and is a promising avenue for improving the efficiency of model training.
However, there would be several technical challenges in applying this approach to large language models. For one, there would be the challenge of how to assess the “understandability” of a text sample in a reliable and useful way. This would likely require significant work to define and might also have to involve some form of reinforcement learning or other feedback mechanisms. Nonetheless, it’s a fascinating idea and could potentially be an interesting direction for future research in machine learning.
I suspect future models are going to have to put some more focus on learning using techniques more like what humans use, and on cognition.
Like, compared to a human these language models need very large quantities of text input. When humans are first learning language they get lots of visual input along with language input, and can test their understanding with trial-and-error feedback from other intelligent actors. I wonder if perhaps those factors greatly increase the rate at which understanding develops.
Also, humans tend to cogitate on inputs while ingesting them during learning. So if the information in new inputs disagrees with current understanding, those inputs are less likely to affect current understanding (there’s a whole ‘how to change your mind’ thing here that is necessary for people to use, but if we’re training a model on curated data that’s probably less important for early model training).
I don’t know details of how model training works, but it would be interesting to know if anyone is using a progressive learning technique where the model that is being trained is used to judge new training data before it is used as a training input to update the model’s weights. That would be kind of like how children learn by starting with very simple words and syntax and building up conceptual understanding gradually. I’d assume so, since it’s an obvious idea, but I haven’t heard about it.
This is why I pay for YT Premium. No way in hell am I watching ads, but I do want to be able to use the platform, and the money has to come from somewhere. So far it’s been pretty good value, although SponsorBlock is of course still required.